Collective Intelligence
(this page is an extract from Deeper-City: Collective-Intelligence-and-the-Pathways-from-Smart-to-Wise – also available as Deeper Mind-PDF
Some of the greatest thinkers in recent history have lived or worked in the UK…. but as of now, its school system is losing teachers, 20% of adults are functionally illiterate and 60% of graduates are under-employed.[i] The UK’s productivity and innovation are well down on its competitors, its people working longer to produce less, with a house value bubble as the centrepiece of the economy. Is it that the UK system is stupid, or just missing its higher potential?
New economic theory stresses the value of ‘learning’, but this seems narrowly defined as functional, competitive and measured by GDP output.[ii] In contrast, synergistic learning looks for cooperation between neighbours, mutual aid of young and old, the creative curiosity of schoolkids, or social design for social justice – a much deeper-wider agenda… So, this section goes right to the heart of synergistic thinking, and asks – what is this deeper mind thing, this collective intelligence – and how to work with it and grow it?[iii]
[i] CIPD, 2015
[ii] Stiglitz & Greenwald 2014
[iii] Terminology note: here we define collective intelligence as a quality of the whole system, and deeper mind as a quality of the various actors or factors within the system (see the Glossary in Chapter 12)
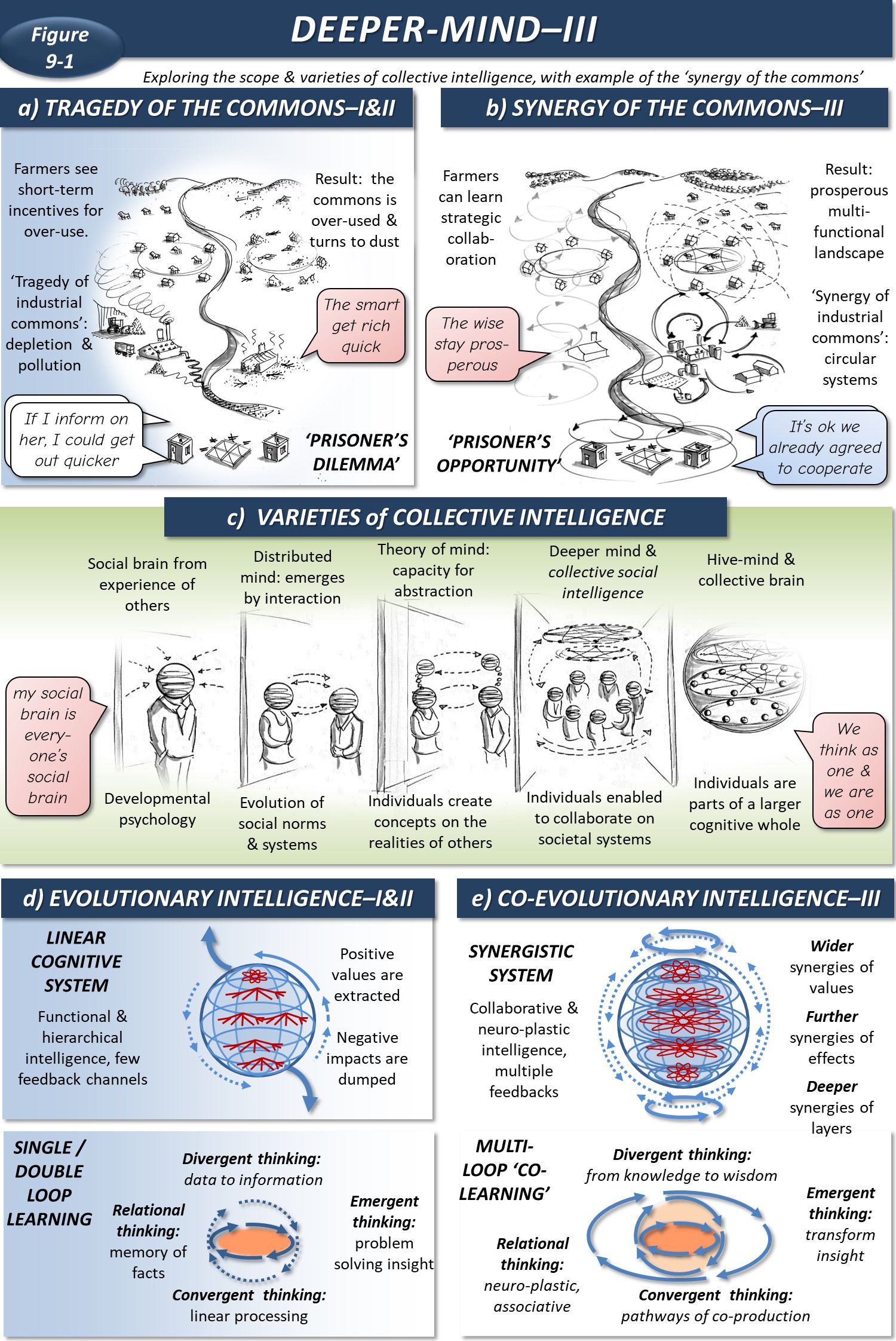
Co-learning curves
Manchester for one, has great aspirations to be a Low-Carb city, but it’s a challenge. It seems that builders need to ‘learn’ about advanced retrofit, financiers ‘learn’ who to trust with loans, suppliers ‘learn’ how their devices perform and residents ‘learn’ about the best deals. Overall, policy-makers, entrepreneurs, institutions of all kinds need to learn ‘what works and how’, in a complex world of risks and opportunities. Way beyond the individual level, this calls for collaborative or mutual co-learning (between people or organizations), and co-creation (shared or networked design thinking). There’s no single theory to explain all this, so here is a brief roundup:
(Box 9a) Overview: learning about learning
From organization studies emerged the ‘double-loop’ learning theory (also providing clues for synergistic thinking):[i] beyond direct functional problems this looks towards wider contextual knowledge, deeper goals and values, and further strategies and intentions. Some push this towards Mode-III or ‘Multi-Modal’ social learning, for collective intelligence which transforms distributed knowledge into sense-making.[ii] This also highlights the difference between ‘tacit’ knowledge versus ‘formal’ knowledge of individuals or organizations.[iii] Another angle comes from developmental psychology, with dual tracks of ‘assimilation’ and ‘adaptation’.[iv] Scaling from the individual level to societal, it seems that social learning (‘co-learning’) is more than a one-way acquisition of knowledge; it’s also moving towards an ‘adaptive fitness’ between social systems and other systems, a ‘collective wisdom’.[v] Learning might also be an evolutionary process for social institutions and their ‘social design,[vi] or with social interactions, modelled in game theory and the mathematics of cooperation.[vii] Complexity economics explores a practical ‘learning by doing’, a ‘deductive tinkering’ as an evolutionary strategy which provides the ‘origins of wealth’.[viii] Meanwhile, the ‘theory of mind’ looks at learning as capacity building, for abstract thinking and inter-personal strategies.[ix]
Two fables come to mind here. One is the ‘tragedy of the commons’, a story of collective action problems, sketched in Deeper-Mind-III (Fig.9-1), upper left a). [x] A community of farmers share the common land, but for each there’s an individual incentive to use more than their share. The land is over-grazed, turns to dust, and the farmers go hungry: locally it’s a problem, but globally it can be catastrophic. Actually, this case rests on a worldview which is assumed as materialistic and myopic. If we look beyond that narrow confine, towards some form of collective intelligence, there’s potential for collaboration, not only from altruism but strategic self-interest. With a deeper-mind kind of logic, the tragedy can be reframed:
<list>
- Social logic: structures of kinship/community, including future generations;
- Technical logic: strategies for integrated land management;
- Economic logic: cost-benefit calculus which includes futurity (with social discount rates), and effectiveness (as with trading and development);
- Ecological logic: principles of stewardship and inter-species respect;
- Political logic: co-creation of institutions of collaboration and cohesion;
- Cultural logic: norms, discourses or kinship systems to support reciprocity and trust.
So, what kind of pathways can lead from a myopic community, towards one of deeper and wider collaboration? If the farmers are locked into material economic logic, then we look for deeper synergies with political or ecological values. If locked in a cultural logic of individualism, then we look for wider social or technical opportunities. And so on, the point being that such a ‘tragedy’ can also become an ‘opportunity’.
Another fable is the ‘Prisoner’s Dilemma’, a landmark thought experiment in game theory. In the storyline, each of two prisoners held in different cells for their part in the same crime has a choice: stay silent, or inform on the other. If both stay silent they each get one year in jail. If both inform on the other, each gets two years. If one informs on the other, the informer gets out free and the other three years.[xi] What should they do? Again, if we assume a myopic individualist mindset, this is a double-bind trap where everyone ends up losing. But with more collaborative and strategic co-learning – with cultural or political or kinship logic – then the prisoners are not only selfish individuals, they are citizens of a community or a culture, and the outcome can be more than a zero-sum game.
Synergistic intelligence
Learning, if anything more than gathering facts, should lead towards some kind of ‘intelligence’, but for such a vital quality, there’s no single view on what it is or how to improve it.[xii] There’s an even bigger gap when it comes to scaling up, from individuals to the collective intelligence of organizations, communities, value chains or societies. This is at the centre of the whole Deeper City concept, but it’s fair to say we are all beginners. First, here are different angles from across the social sciences:
(Box 9b) Overview: thinking about thinking
Cognitive sociology looks at mental processes that combine for the experienced ‘realities’ of individuals and communities, such as perception, attention, classification, association, memory, and time reckoning.[xiii] The ‘Shared Mind’ proposition challenges the ‘theory of mind’ proposition, suggesting that the human mind is based on intersubjectivity and the interpersonal sharing of experiences.[xiv] Evolutionary anthropology looks at historic evidence on the role of cognition in behaviour, which combines the ‘social brain’ and ‘distributed mind’ concepts.[xv] Evolutionary psychology looks at the evolution of the social mind to understand schizophrenia and other pathologies.[xvi] An evolutionary systems approach shows up with the ‘self-organizing social mind’ view of human relations.[xvii] Sociology and urban studies have long considered the evolution of social institutions as collective conscious collaborative projects.[xviii] And social psychology is now looking at group intelligence as ‘group problem solving’: one recent study found correlations between collective intelligence, social skills and gender balance.[xix] This also relates to the notion of fluid versus crystallized intelligence: ‘fluid’ being the capacity for creative thinking on novel problems, while ‘crystallized’ depends on prior knowledge of the problem.[xx] ‘Neuroplasticity’ also looks at the flexibility and self-organization of the individual brain, with parallels at the collective level. All this works not only on the level of human brains, but between ‘actors and factors’, humans and technologies, as in the concepts of ‘extended mind’ and ‘distributed cognition’.[xxi]
Drawing on these many strands, the cartoon shows a simple spectrum in the centre of Deeper-Mind-III, reading from left to right:
- ‘Social brain’: individual cognitive knowledge and skills, formed with influence from others;
- ‘Distributed mind’: emerges by inter-personal social exchange and mutual understanding;
- ‘Theory of mind’: the capacity for abstraction of the mind of others;
- ‘Deeper-mind’: a component of a collective intelligence, as discussed throughout this book (some refer to a similar idea as shared mind). This focuses on the synergies which enable individuals to collaborate on societal systems. The intelligence here is both in the collective system structures, feedback channels, creative design capacity, and in the individuals who participate within it. Such collective intelligence may be enhanced by AI, or with digital-human combinations of
- At the far end of the spectrum is the ‘hive-mind’, a super-brain where individuals are subsumed in a larger whole, with the image of worker bees. Such systems may yet emerge in digital-human combinations of ‘super-intelligence’, as pictured by Informatics-III (Fig.7-2).
Deeper forms of intelligence
In many walks of life it’s now accepted that intelligence is much more than technical ‘know-what’. ‘Emotional intelligence’ is essential in business and other organizations (and a favourite of the self-help manuals).[xxii] Cultural intelligence is vital in media and creative arts. Ethical intelligence helps to manage business risks. A useful mapping comes from Gardner’s theory of ‘multiple intelligence’, which include ‘logical-mathematical, spatial, linguistic, bodily-kinaesthetic, musical, interpersonal, naturalistic and existential’.[xxiii] (It’s interesting that music is a category on its own). This portfolio is broadly similar to the synergistic menu of (so-called) ‘STEEPC’ domains. So, here’s a starter menu for the deeper layers, sketched in Deeper-Mind-III (Fig.9-1) lower section e) and f):
- Social intelligence, or ‘know-who’: networking and inter-personal skills, with social/emotional intelligence, for empathy or social network capacities;
- Technical intelligence, or ‘know-what’: functional problem-solving, with adaptation and innovation;
- Economic intelligence, or ‘know-how’: entrepreneurial or practitioner skills, as in business, enterprise, craft work or professions;
- Ecological intelligence, or ‘know-where’: biological and ecological intelligence, for life-support balance with inter-species connectivity;
- Political intelligence, as in ‘know-who/how’: strategic and leadership skills, for political ‘nous’ in setting agendas, managing institutions, mobilizing resources;
- Cultural intelligence, or ‘know-why’: creation of meaning, significance, identity, morality, with practical intuition for creative arts, sports, everyday social exchange.
This menu is the start of an exploration of pathways from smart evolutionary intelligence, towards a wiser co-evolutionary intelligence. In contrast to the version on the lower left d) – hierarchical, extractive, dysfunctional and basically unsmart – this connexus of collective intelligence on the lower right e) of Deeper-Mind-III, looks like a multi-inter-connected crystal ball. It shows positive values re-circulated, and negative impacts internalized. It shows a general structure of deeper vertical links, wider connections and further lateral feedbacks.
If we zoom into one section of this crystal ball, it looks just like the model of Enterprise-III (Fig.5-4), where parallel value chains link upstream-downstream with a collective economic intelligence. Other pathway models also track onto the same basic landscape: Smart-Services-III (Fig.7-3) connects the value chain with digital efficiency, Organization-III (Fig.8-3) is about the vertical links between layers, and so on. The Multi-versity-III (Fig.10-4) then translates this concept structure into a real-world Collaboratorium, with practical ways of running debates, enquiries, action research, synergistic mapping and design.
‘Wider’ and ‘further’ intelligence
Turning the ‘tragedies of the commons’ into ‘opportunities’, pictured on the top right b), it seems quite practical for farmers to learn about co-production, or for factories to learn that environmental management is good for business. In this it’s clear that different parts of society, public or private or civil, each have different mental models and frames. In this wider sense, knowledge is not only data or information, but the means to its mobilization and value and significance. As pictured in the Multi-Versity-III, this was set out by Aristotle as ‘phronesis, episteme, techne’, or in modern equivalents: ‘societal know-why, scientific know-what, and practical know-how’. For the Low-Carb city example, or any similar challenge, this calls for all the collective low-carb intelligence we can throw at it, technical and economic, social or political or cultural. And in each of these we see the different Modes, working in parallel
- Linear (Mode-I) intelligence, for direct problem-solving: for example, matching energy technology to the right house type and finance package;
- Evolutionary (Mode-II) intelligence: more about creative enterprise, such as energy markets, social incentives, technology innovation or creative cultures.
- Co-evolutionary (Mode-III) collective intelligence: this brings together technology, people, organizations, governance, finance, supply chains and so on, for the bigger picture. Either this happens by accident, or by strategic intention. Some would frame this as ‘strategic policy intelligence’, others as social design thinking. We could also frame this as the venerable word, ‘wisdom’. And the pathway between ‘smart’ (winning an argument with your partner) and ‘wise’ or at least ‘wiser’ (knowing when not to argue!) is crucial.[xxiv]
Practical questions arise for the Low-Carb city: how to measure or manage this vital quality of collective low-carb intelligence? How to improve or enhance it? Such questions point towards a process rather than a fixed answer.
‘Circulatory’ process and dynamic intelligence
In many ways this intelligence concept is more useful, not as a static condition to be measured, but a dynamic ‘process’ of collaborative (co-) learning and thinking, which can be enabled or catalysed. Pictured in Deeper-Mind-III (Fig.9-1), lower left corner d), is a four-part cycle of ‘single / double loop learning’. This starts with relational ‘know-who and how’ thinking, then goes to divergent ‘what-if’ thinking. It moves to emergent ‘why-not’ thinking, and then convergent ‘so-what-to-do’ thinking.[xxv] These four stages match with the Synergistic Toolkit and its cycle of ‘4-S’ (systems/scenarios/synergies/strategies). They also look similar to another landmark in the field, Sternberg’s ‘Triarchic’ theory of intelligence.[xxvi] This starts with ‘componential’ intelligence, meaning ‘performance’ (memory and problem-solving) and ‘knowledge’ (information gathering and filtering). There follows the ‘experiential’ intelligence, with so-called ‘giftedness’ or ‘novelty’ (creativity and innovation), and ‘automation’ (efficiency of learned patterns and routines). The final strand here is the ‘mobilization’ to shape external situations by practical action.
And then the broad contours of a synergistic collective societal intelligence start to emerge on the lower right e). The dynamic process is that of ‘multi-loop co-learning’. Here there is not one domain of logic, but many (social, technical, economic, etc). The cognitive functions are more fluid, associative, distributed and neuro-plastic. The ‘giftedness’ works by co-creation and leaps of collective imagination, and the mobilization and solutions work by co-production of all the parts in synergy. The result is then visualized as a ‘synergistic system’ with the crystal ball image, which contains deeper synergies between layers, wider synergies between actors, and further synergies between cause-effect chains. In other words, the mapping of a collective intelligence seems to resemble its results, i.e. the systems which can be created or managed by that same collective intelligence. This could be very significant for the design of intelligent finance, political decision-making, industrial supply chains or others: in each case, the system architecture or organogram can resemble the mapping of the collective intelligence behind it. This might all sound a little abstract, but the applications are very practical. A wiser organization (i.e. one with collective organizational intelligence) has active feedback channels to learn and remember and think ahead. A wiser local economy (with collective economic intelligence) provides the mututal information, the mutual learning of the players, the synergies between them, the creative energy for innovations, and so on. And for our Low-Carb city, we look for a collective low-carb intelligence, so all actors can learn, think ahead, form ideas and act on them in the complex mix of energy firms, construction, residents, designers, investors and inventors and many others.
Systems intelligence and consciousness
Further on the trail of intelligence and consciousness or ‘psyche’, there’s an emerging nexus. It seems there are vital crossovers between neurology, AI and robotics, evolutionary biology and complex systems. One of the closest to synergistic thinking is the ‘Integrated Information Theory’ (IIT) of consciousness.[xxvii] This tracks the system conditions for cognitive learning and processing: from an amoeba to an insect, from a human to a civilization, the conscious intelligence of any system can (in principle) be framed in terms of cognitive complexity. From any particular brain, with its neurons and axons, dendrites and synapses, it looks for the degree of integration, with ‘the size of the conscious repertoire associated with any network of causally interacting parts’. In other words, the number of parts and potential links between them, the number of channels and potential formations in learning/thinking/sense-making, all add up to a cognitive complexity. With synergistic thinking we can build on the IIT proposition. The neural system, whether individual or city or civilization, can look to deeper layers beyond the functional: it can look wider to all actors and factors in the system, and then further upstream/downstream of its immediate scope or problem.
This follows on from the ‘wisdom of crowds’ and earlier ideas on techno-utopia (the ‘wisdom’ here being more or less Mode-I type knowledge).[xxviii] If the ‘crowd’ is a randomized set of individuals, like molecules in motion, then algorithms to gather the aggregated knowledge or judgment (i.e. ‘crowd-wisdom’) can work well. But if the crowd is highly self-organized, with conscious reflexive feedback, it could be more like the IIT hypothesis of consciousness. The tools for gathering knowledge are integral to the result (election polls which influence the result), with results depending on social feedback (online Delphi) with outcomes which are highly volatile (financial trading which shapes the market).[xxix]
So – if a synergistic Low-Carb city policy or programme, such as the Retrofit-III (Fig.4-4), is responsive and self-organizing, does this mean it has real intelligence and/or consciousness? The short answer is, not necessarily! There are questions of ‘scale’ and ‘optimality’ and ‘enablement’ of sub-systems. One very important sub-system, the human brain, has something in the order of 1010 cortical neurons and 1080 possible neural pathways (a magnitude similar to the atoms in the known universe). By contrast a Low-Carb city programme might have at most 102 nodes and 103 neural pathways, in the form of project management options or supply-chain links, and the simplest micro-organism is vastly more sophisticated than this. With AI we could design a more intelligent programme or platform, not ‘conscious’, more like ‘optimal’. This could aim to enable humans to improve their homes, not only as objects but as liveable homes, in the sense of an ‘optimal’ retrofit. In reverse, a stupefying or ‘unsmart’ housing programme can easily be sub-optimal even if it works, with energy wastage, fuel poverty or welfare system traps (for instance in Public-Service-III, Fig.8-4).
How to tell the difference?? If the low-carb algorithms work with known data and standard rules, they could succeed as a ‘clever’ system. If data is missing, the rules are fluid and the residents are in competition then we need more of a ‘smart’ system. If there are deeper or wider issues of social justice, urban regeneration or longer term investments, then we need something more ‘wise’. New challenges come with digital systems, already moving from ‘smart’ cities to (so-called) ‘conscious’ cities, which are beginning to simulate emotional or cultural experience. As in Informatics-III (Fig.7-2), the potential is huge, but there’s an open question whether technology will empower the humans or enslave them. With huge resources going into AI, it’s all the more urgent to explore the human side of the CHAI (Collective Human-Artificial Intelligence).[xxx] For instance, a near-future city of 10 million people each with 100 IoT feeds has potentially 109 nodes as a baseline for a smart AI system, as in Smart-Communities-III (Fig.7-4). By default such an AI will tend to prioritize the functional systems of measurable production-consumption, just as Google and other platforms privilege their clicks and views. For any alternative systems of co-creation and co-production, or alternative structures of socio-economic power or status, it’s the role of the combined CHAI to realize the potential for emerging wider consciousness. These are generally experienced at certain moments, such as in Manchester after the 2017 terrorist attack (Societal-III, Fig 10-1): the question is then how to sustain and grow from there?
Practical collective intelligence
So now, the trillion-dollar question – what’s the collective intelligence, or deeper-mind, or cognitive capital of our city, organization, business, policy, project, or product? And how to grow or improve it? Intelligence testing for individuals has in the past promoted discrimination or even eugenics, and for the most part has ignored any collective dimensions. Any method of ‘collective intelligence testing’ could also be controversial. But for any human endeavour, from a corner shop to a global economy, there’s an urgent need to understand and track this vital quality.
Many examples through this book show this. The Green Deal policy in Retrofit-III (Fig.4-4) was a lesson in failure, a basically un-smart system of financial hurdles, information gaps and institutional divides. In contrast, the Global Forest Watch from Climate-III (Fig.6-3) seems (so far) successful, with positive synergies between the technology and climate policy, business and local communities.
The summary and self-assessment here (Table 9-1), is a simple way to begin exploring the cognitive capital of any system, large or small, working in Modes-I, II or III. For each it asks some basic questions:
- First, the functional Mode-I ‘clever’ qualities: what level of functional inputs, information processing, decision outputs and action outcomes? Can the system respond to ‘known known’ problems, and decide on effective solutions?
- Similar for the evolutionary Mode-II ‘smart’ intelligence: what level of cognitive capital to deal with incentives, enterprises, competitions, innovations? Can the system respond to new problems or opportunities (or ‘unknown knowns/known unknowns’)?
- Similar for the co-evolutionary Mode-III co-intelligence: how does the conscious complex system work, for the wider community of actors, and the deeper layers of value? Can the system respond to emergent problems as part of a larger community? Is each stage of the ‘circulatory’ cognitive cycle effective, for co-learning/co-knowing/co-creation/co-production? Can the system respond to ‘unknown unknowns’?
Table 9-1: Deeper-Mind-III: summary & self-assessment
|
Mode-I Linear |
Mode-II Evolutionary |
Mode-III Co-evolutionary |
|
‘CLEVER’: complex |
‘SMART’: emergent complexity |
‘WISE’: Deeper complexity |
DEEPER: (domains, layers) |
Mono-functional, technically clever |
Multi-layer, multi-function, competitive-innovative |
Combined emotional, ethical, cultural intelligence |
WIDER: (actors) |
Individual actor: person brain power |
Some actors: team, firm, project, supply chain |
All actors: community, network, society |
FURTHER: (factors) |
Direct tangible links only |
Strategic upstream/downstream links |
Whole system upstream/downstream links |
CIRCULAR: (process) |
Functional intelligence |
Human intelligence |
Collective intelligence |
Relational thinking
|
Memory of facts |
Learning |
Co-learning |
Divergent thinking |
Information |
Knowledge |
Co-knowledge/sense-making |
Emergent thinking |
Solution to problem |
Design for opportunity |
Co-creation for transformation |
Convergent thinking |
Plan |
Strategy |
Co-production/co-innovation |
This matrix is not the end but the beginning of a question. It’s possible to find numbers for each row and column, for any particular problem or project or organization. However, until there’s a common agreement on a CIQ (‘Collective Intelligence Quotient’), it’s not practical to use the numbers for comparison or benchmarking. We could refer back to the IIT theory above, but this has a rather major flaw, impossible (at present) to calculate for all but the simplest cases. In practice a range of indicators or benchmarks can be useful in combination with a deliberative enquiry and dialogue.
It’s useful to compare with parallel systems, such as the SEI ‘emotional intelligence’ tool, which contains three general ‘pursuits’, eight competencies and 18 practical ‘brain apps’.[xxxi] The equivalent version for synergistic collective intelligence starts with the framework in the summary (Table 9-1): deeper, wider, further, circular, with three system modes. Then it explores all possible pathway combinations, of social/ technical/cultural/economic and so on (Table 12-6 in the Annex). Overall, there may never be a single agreed concept of collective intelligence, or an index for measuring it, but we can use the assessment in a creative process of mapping and design. And just to be clear, any search for data raises the question of ‘what is to be measured’: whether internal, procedural or external:
- Internal: cognitive capacity of the collective intelligence, via measures of learning and thinking, or systems feedback and processing (e.g. the cognitive quality of a housing system, public or private);
- Procedural: system activities or interchange, which applies the internal capacity to the external results (as seen in housing policies, investment, innovation etc);
- External: results or outcome of action based on collective intelligence; easier to observe but subject to contingent factors (housing which is liveable, prosperous, resilient etc).
Similar issues come up in many of the global league tables (but usually not in a transparent way): some appear in the pilot Scanning Tool (see Developmental-III, Fig.10-2, and online). This collects indicators for each domain and each Mode of a Deeper City, visualized in a spider diagram, not as an end but a start of discussion (Table 12-8). In principle this can shed light on any component of a Low-Carb city – firms, sectors, infrastructures, taxes, policies or innovations – or any combination of these. And this leads to the next pathway on the journey, the perennial question of ‘value’…
[i] Argyris & Schön 1996; Elia & Poce 2010
[ii] Tosey, Visser & Saunders 2012
[iii] Nonaka & Toyama 2005
[iv] Piaget & Inhelder 1973
[v] Landemore & Elster 2012
[vi] Ostrom 2005
[vii] Axelrod 1984
[viii] Beinhocker 2006
[ix] Apperly 2010
[x] Hardin 1968
[xi] Axelrod 1984
[xii] Terminology note: ‘business intelligence’ or ‘military intelligence’ have more specific meanings, as in covert or asymmetric information: ‘collective intelligence’ is also used to mean digital social media information/exchange. Here we use ‘collective intelligence’ in its wider sense. Also note the Institute for Co-Intelligence uses ‘co-intelligence’ (see the Glossary in Chapter 12)
[xiii] Zerubavel 1999
[xiv] Zlatev et al 2010
[xv] Malone & Bernstein 2015
[xvi] Siegert & Ward 2003
[xvii] Bolender 2010
[xviii] Dewey 1939
[xix] Woolley et al 2010
[xx] Cattell 1971
[xxi] Clark & Chalmers 1998
[xxii] www.6seconds.org
[xxiii] Gardner 1993
[xxiv] Kaipa and Radjou 2013
[xxv] Ratcliffe & Krawczyk 2011
[xxvi] Sternberg 1985
[xxvii] Tononi & Koch 2015
[xxviii] Mulgan 2016
[xxix] Von Foerster 2003
[xxx] Palti & Bar 2015
[xxxi] www.6seconds.org